What federal agencies can learn from New York City’s AI hiring law
As the Biden administration begins implementing its executive order on artificial intelligence and federal agencies start to make critical decisions about how to create fair frameworks around their use of AI, one recent law offers important lessons about what it will take for them to be effective and protect civil rights.
That law, New York City’s Automated Employment Decision Tool law — Local Law 144 — is the first to require algorithmic fairness audits for commercial systems. Under Local Law 144, employers must notify anyone who applies for a job located in NYC if they are using certain AI-powered automated employment decision tools in the process of hiring or promotion. Additionally, these employers must annually conduct and publicly post independent audits to assess the statistical fairness of their processes across race and gender.
The operating theory of the law is that if employers are forced to measure and disclose the fairness of their algorithmic hiring and promotion systems, they will be incentivized to avoid building or buying biased systems. Researchers and investigative journalists have shown repeatedly that AI systems like these — which rank applicants based on an automated analysis of factors that can range from their resume to their perceived emotions in a video interview — can produce biased results.
Evidence shows that many automated systems were trained on data that reflects human, historical biases, and/or have been developed based on spurious correlations. Although federal agencies have indicated that automated systems must follow existing civil rights laws prohibiting discrimination, New York’s law is the very first regulation to mandate that anyone developing or deploying these systems share evidence about the fairness of their decisions with the public.
Yet early studies conducted by my research collaborators and I have found that compliance with the law appears to be shockingly low. Importantly, our research shows that at least part of the issue is with the law itself: Despite good intentions, the way the law structures accountability makes it functionally impossible to determine whether the absence of an audit indicates non-compliance and why the non-compliance is occurring.
Ostensibly, any covered employer or recruitment firm must place their audits somewhere online where the general public — not just job seekers — can find them, typically on their available jobs or human resources page. Automated employment decision tools are very widely used today; indeed, it is now rare that job applications are not subjected to some type of AI. But if you go looking for examples, you’ll likely be disappointed.
Local Law 144 did not create a central repository for these audits, which means finding them requires going through potential employers one by one. With dozens of hours of professional researcher labor since the law went into effect in July, my collaborators and I have found fewer than 20, and some of those have already disappeared from public view.
What explains this? Is the absence because an employer doesn’t use any algorithmic systems, or because they think the law does not cover their algorithmic systems? Unfortunately, Local Law 144 does not demand the level of transparency that could reveal more. Yet our research holds clues: In interviews, independent auditors have consistently indicated that clients of theirs have paid for the mandated audits but ultimately chose not to make them available to the public.
There is enough variety in the ways these systems are built and operate that definitions matter dramatically. Local Law 144’s definition of machine learning, and its vague criteria for systems that “substantially assist or replace human decision-making” offer enough wiggle room to be creatively lawyered around. Future regulations would benefit from broader definitions that are scoped around the purpose or use of the systems, rather than their technical specifications.
Similarly, the relationship between platforms, vendors, end-user employers and auditors must be addressed directly to clarify lines of accountability. Local Law 144 depends on auditors having adequate access to the backends of the systems that vendors sell to employers, but the law does not address vendors or their contractual conditions at all. It places obligations only on the employer using the system to assist hiring decisions and leaves it up to them to use market pressure to get access to, and presumably change, the vendors’ systems.
Furthermore, the law’s emphasis on decision-making lets recruiting platforms like Indeed, LinkedIn and Monster largely off the hook. Though they are the gorillas in the industry and use algorithms to measure, rank and distribute every job-seeker, they do not render any final hiring “decisions” (Indeed explicitly states as much), and therefore fall into an accountability gray area that Local Law 144 and other employment laws do not address.
Another lesson is the importance of aligning regulations and incentives across layers of the government. Employment anti-discrimination law is notoriously complex, with extensive judgment calls and rules of thumb, and few hard standards amenable to the quantitative nature of AI audits. This means that if an employer or vendor conducts an audit — either for compliance with a local jurisdiction or for pursuing a market advantage — they may be disclosing evidence of biased decision-making that draws the attention of powerful regulators or private litigators.
Notably, Local Law 144 does not set a threshold for acceptable rates of bias (aka “disparate impact”) for sale or use. The Equal Employment Opportunity Commission also deliberately avoids naming just such a hard statistical line between acceptable and unacceptable bias for AI systems, but it also has not provided any guidance about safe harbors for audits conducted in good faith.
Given those conditions, it’s no surprise that some employers are paying for audits but not publishing them, undermining Local Law 144’s good intent. Without safe harbors and/or clear guidance about permissible levels of statistical bias, employers will be stuck between competing pressures and ambiguous standards, and routine auditing will not occur across the industry.
Finally, researchers and regulatory agencies can only study the patterns of bias in these systems if investigators can actually find the audits, and job seekers can only make informed decisions about their applications if the audits are readily available. Future regulations should require that audits be put in a central repository that anyone can access, or else enforcement will be ad hoc and egregiously time-consuming.
We often assume that when there is a new obligation to assess something, the ecosystem to do so will grow up around it and eventually exercise “soft power” to incentivize good behavior. However, the shortcomings of Local Law 144 so far show that this is not a given.
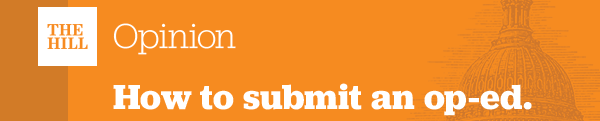
Algorithmic tools are like airplanes: They require a large ecosystem of mutually accountable people and institutions to keep them operating safely. Local Law 144 operates as if the Federal Aviation Administration wrote rules only for pilots, hoping that the pilots would then demand sound aeronautic engineering and competent air traffic controllers.
Effective regulations for algorithms must instead be grounded in distributed accountability, where everyone involved is required to transparently account for the system’s safety and fairness. Such expectations are aligned with those we hold for areas as different and essential as air travel and civil rights and are fitting for the automated tools that stand to impact so many areas of our lives.
Jacob Metcalf is program director of AI on the Ground at Data & Society.
Copyright 2023 Nexstar Media Inc. All rights reserved. This material may not be published, broadcast, rewritten, or redistributed. Regular the hill posts
Video/Hill.TV
