To promote AI effectively, policymakers must look beyond ChatGPT

The emergence of ChatGPT — which reached 100 million active users just two months after launch — has everyone talking about artificial intelligence (AI), including lawmakers in Washington. Senators and the Biden administration have already expressed concern about the disruptive potential of new AI technologies.
Between academia’s reservations about the impacts of these technologies, Chinese companies’ attempts to roll out competing models and worries about new AI models being used to supercharge influence operations, policymakers seem to agree that urgent action is necessary. Although a major policy focus to date has centered on computing power, or “compute,” a new study from Georgetown University’s Center for Security and Emerging Technology (CSET), where I am a research analyst, suggests that these tactics may be less effective than many researchers and policymakers have assumed.
It is true that some modern AI systems are notoriously compute-intensive, requiring rapidly growing expenditures of computing power to train. You may have seen graphics in leading publications showing an explosion of computing power used to train leading AI models. Many commentators increasingly worry that this dependency on expensive hardware is creating a “compute divide” between industry and academia and that academia risks being left behind as industry jumps ahead in AI research.
At the same time, if modern AI is so dependent on computing power, maybe that makes it a good leverage point for policymakers. In October, the Biden administration launched new export controls on high-end graphics processing units (GPUs) and related technologies flowing to China, in large part to prevent further development of advanced AI capabilities. And in January, the National AI Research Resource (NAIRR) Task Force released its final report outlining how the U.S. can promote AI research and reduce divides between industry and academia: fund a centralized resource for researchers, and reserve the largest expenditures “for large computing investments.”
But this thinking may be missing the bigger picture, as new survey research from CSET reveals. In this work, we asked more than 400 AI researchers from across the United States how much computing power they use in their work, how worried they are about having insufficient compute for future projects and how important they think it is for AI progress generally.
What we found surprised us: When respondents changed their research plans, it was more often because they lacked data or talent than because they lacked computing power. If they had more money to spend, a majority said they’d spend it on hiring more talent, with only a fifth indicating more compute as their top priority. We also found that academics were not significantly more likely than industry researchers to express concern that they lacked enough compute to make meaningful future contributions to their field. In fact, most academics reported using levels of compute for their past projects that were surprisingly consistent with the amounts used in industry.
Perhaps these results shouldn’t have been surprising. Most of the graphs showing an explosion in the compute needs of AI models draw from a single database of “notable” models; of the most recent 25 models with compute information deemed “notable” enough to include in this dataset, about 75 percent are language models. But it’s certainly not the case that three out of every four AI researchers focus on language modeling. What these figures really show is an explosion of compute needs within a specific sub-topic in AI research, and while language models like ChatGPT are certainly impressive, there are many other types of research projects we ought to be pursuing.
As just one example: Some research suggests that “AI safety” may make up only 2 percent of all AI research being conducted today. Safety research — which can study topics like adversarial attacks on AI systems or the interpretability of AI models — isn’t necessarily all that compute-intensive. And yet, in their rush to quickly deploy large language models, companies are shirking this type of work. This is a clear area where government support for greater research could make a difference.
If Congress decides to fund the NAIRR, it would be an enormous missed opportunity to simply purchase a large amount of hardware and replicate the compute-intensive language modeling that industry is already doing, instead of thinking carefully about how to promote other types of research that are currently being sidelined.
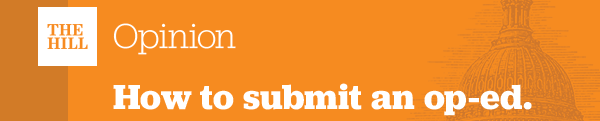
In addition to broadening a national research agenda, our survey results make clear that the biggest constraint facing many AI researchers today is access to talent, not access to hardware. In the medium term, educational initiatives and workforce development programs could help address this problem, and in the short term, increasing caps for skilled immigrants could go a long way toward helping universities and companies alike get the talent they need.
Policymakers may be hesitant to pursue these interventions because — unlike buying a lot of GPUs or preventing others from doing so — they can be politically charged or slow to bear fruit. But AI commentators need to make sure that their policy proposals are actually responsive to the AI field as a whole, and not just to a few important but anomalous language models.
Micah Musser is a research analyst at Georgetown University’s Center for Security and Emerging Technology (CSET) where he works on the CyberAI Project, and the lead author of “‘The Main Resource is the Human’: A Survey of AI Researchers on the Importance of Compute.”
Copyright 2023 Nexstar Media Inc. All rights reserved. This material may not be published, broadcast, rewritten, or redistributed. Regular the hill posts